OME-Zarr
Prerequisites
Before starting this lesson, you should be familiar with:
Learning Objectives
After completing this lesson, learners should be able to:
Understand the OME-Zarr image file format
Render cloud (S3 object store) hosted OME-Zarr image data
Access the pixel values of cloud hosted OME-Zarr image data
Apply basic image processing on cloud hosted OME-Zarr image data
Motivation
Storing TB-sized image data locally and in multiple copies is either not possible or inefficient. Cloud storage enables efficient concurrent access to the same image data by multiple clients (scientists). OME-Zarr is the emerging community standard image file format for cloud (S3 object store) compatible image data storage. Thus it is important to know how to access S3 hosted OME-Zarr in various image analysis and visualisation platforms.
Concept map
Figure
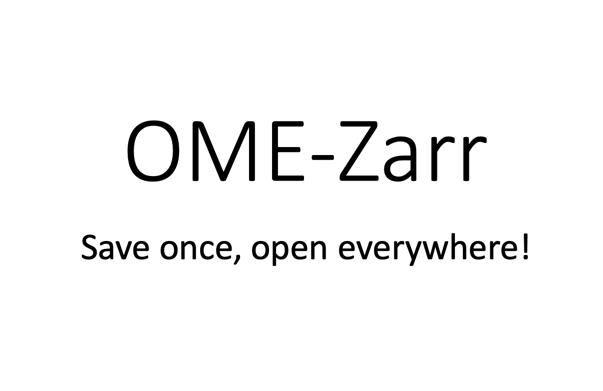
Activities
OME-Zarr inspection
Inspect the OME-Zarr layout and metadata.
Show activity for:
minio-client
Check out what we have at our s3 bucket:
mc tree -d 2 s3/ome-zarr-course/
mc ls s3/ome-zarr-course/data/MFF/
mc ls s3/ome-zarr-course/data/JPEG/
mc ls s3/ome-zarr-course/data/ZARR/common/
Check out the multiscales metadata for one of the OME-Zarr datasets we created:
mc cat s3/ome-zarr-course/data/ZARR/common/13457537T.zarr/.zattrs
Check out the array metadata for the highest resolution array:
mc cat s3/ome-zarr-course/data/ZARR/common/13457537T.zarr/0/.zarray
Configure mc for anonymous access to public s3 buckets:
mc alias set s3pub https://s3.embl.de
Have a look at the metadata for a big OME-Zarr data:
mc cat s3pub/i2k-2020/platy-raw.ome.zarr/.zattrs
mc cat s3pub/i2k-2020/platy-raw.ome.zarr/s0/.zarray
ome-zarr-py
Use the ome_zarr tool for the inspection:
ome_zarr info https://s3.embl.de/ome-zarr-course/data/ZARR/common/13457537T.zarr
ome_zarr info https://s3.embl.de/i2k-2020/platy-raw.ome.zarr
OME-Zarr read performance
Measure how fast you can read OME-Zarr chunks.
References:
- https://forum.image.sc/t/benchmarking-ome-zarr-s3-access/102262
Show activity for:
vizarr Chrome
- Open the Google Chrome webbrowser
- Open the below web address to launch vizarr to visualise an OME-Zarr image
https://hms-dbmi.github.io/vizarr/?source=https://s3.embl.de/yeast-clem/hela/images/ome-zarr/em-overview.ome.zarr
- Note that you may replace the part after
?source=
with another OME-Zarr URL that you may be interested in testing.- Google Chrome: [ View > Developer > JavaScript Console ] and then choose the “Network” tab.
- Now, in vizarr, move around, e.g., zoom in and out; this should trigger the loading of additional OME-Zarr chunks, which can be observed in the Network tab, where you can see how large each chunk and how long it took to read it.
- Typical, in our experience, is a few hundred ms for a few hundred KB.
OME-Zarr creation
Create OME-Zarr data from data stored in monolithic file formats.
Show activity for:
BatchConvert
First check out what data we have the s3 end:
mc tree -d 2 s3/ome-zarr-course/
There are multiple conversion modes. Let’s try each of them.
Perform parallelised, independent conversion:
batchconvert omezarr -st s3 -dt s3 --drop_series data/MFF data/ZARR/$USER;
This command maps each input file in the
data/MFF
folder to a single OME-Zarr series, which is then transferred to a user-specific folder. Note that the-st s3
option makes sure that the input path is searched for in the s3 bucket, while-dt s3
triggers the output files to be transferred to the s3 bucket under the output path.Perform grouped conversion:
batchconvert omezarr -st s3 -dt s3 --drop_series --merge_files --concatenation_order t data/JPEG data/ZARR/$USER;
This conversion mode assumes that the input files are part of the same series and thus will merge them along a specific axis during the conversion process. The
--merge_files
flag specifies the grouped conversion option and the--concatenation_order t
option allows the files to be merged along the time channel.Check what has changed at the s3 end after the conversion:
mc tree -d 2 s3/ome-zarr-course/
mc ls s3/ome-zarr-course/data/ZARR/$USER/
Optional: Copy the converted Zarr data to the home folder:
mc mirror s3/ome-zarr-course/data/ZARR/$USER ~/data/ZARR;
Open OME-Zarr in Java ecosystem
Open OME-Zarr data in the Java ecosystem.
- Intensity multi-scale image:
https://s3.embl.de/i2k-2020/platy-raw.ome.zarr
- Label mask multi-scale image:
https://s3.embl.de/i2k-2020/platy-raw.ome.zarr/labels/cells
Show activity for:
Fiji/MoBIE
- Open Fiji with MoBIE
- [ Plugins > MoBIE > Open > Open OME ZARR… ]
Image URI
:https://s3.embl.de/i2k-2020/platy-raw.ome.zarr
( Labels URI )
:https://s3.embl.de/i2k-2020/platy-raw.ome.zarr/labels/cells
( Labels Table URI )
: TODO
Fiji/n5-ij-viewer
Open a remote OME-Zarr in Fiji
- Open the n5-ij in Fiji via:
[ File > Import > HDF5/N5/Zarr/OME-NGFF ... ]
- In the window that opens, paste the following path in the uri space:
https://s3.embl.de/ome-zarr-course/data/commons/xyz_8bit_calibrated__fib_sem_crop.ome.zarr
Then click
Detect datasets
button as shown below:The tool will display a multiscales schema with two datasets in the dialog box. Select one of the datasets as shown below and click OK:
- This will open the dataset in Fiji as a normal Fiji image (see below).
Open a subset of a remote OME-Zarr in Fiji
Follow the same steps above do select a dataset but instead of directly opening the dataset, click the crop button in the window before clicking OK as shown below:
In the window that open, select the indices of the subset as shown below:
When you click OK, the specified subset of the image will be opened as shown below:
Open a remote OME-Zarr in BigDataViewer
Now let’s imagine the dataset you want to open is too large to fit the RAM of your machine.
- Open the n5-viewer in Fiji via:
[ Plugins > BigDataViewer > HDF5/N5/Zarr/OME-NGFF Viewer ]
- In the window that opens, paste the following path in the uri space:
https://s3.embl.de/i2k-2020/platy-raw.ome.zarr
Then click
Detect datasets
button as shown below:
The tool will display a multiscales schema with 9 datasets in the dialog box. In this case, one can either open the individual datasets or the entire pyramid. To do the latter, click on the multiscale object and then click OK as shown below:
This will open the multiscales object in BDV as shown below:
This is a huge (terabyte-scale) image, which is not amenable to processing as a whole in Fiji. It is possible, however, to extract subsets of it to Fiji and continue with processing. To do so, follow the steps below:
- In the BDV window, open the cropping window via:
[ Tools > Extract to ImageJ ]
(also see below)
- In the cropping window that opens, select the indices of the subset as shown below:
Note that this step may require incremental rotation of the image and adjustment of the bounding box until the desired region of interest is obtained. It is also important to check the size of the cropped volume at the top of the cropping window to make sure that it is not larger than the memory. Once you are fine with the settings, click OK.- The output is a standard Fiji image as shown below:
Note that this image has been loaded into the RAM; as such, it can be processed like any other Fiji image and saved to any desired file format.
OME-Zarr visualisation
- Visualise S3 hosted OME-Zarr images
- Appreciate that the data can be multi-scale and chunked
- Example data:
- URL: https://s3.embl.de/ome-zarr-course/data/ZARR/$USER/xyzct_8bit__mitosis.ome.zarr
- Content: Small fluorescence microscopy image. Just replace $USER with your user name.
This dataset is one of the outputs from the “ome_zarr_creation.md” activity
- Content: Small fluorescence microscopy image. Just replace $USER with your user name.
- URL:
https://s3.embl.de/i2k-2020/platy-raw.ome.zarr
- Content: 3-D EM with label mask
- Metadata: https://s3.embl.de/i2k-2020/platy-raw.ome.zarr/.zattrs
- URL: https://s3.embl.de/ome-zarr-course/data/ZARR/$USER/xyzct_8bit__mitosis.ome.zarr
Show activity for:
napari
Visualise the remote data using Napari together with the napari-ome-zarr plugin.
napari --plugin napari-ome-zarr https://s3.embl.de/ome-zarr-course/data/ZARR/$USER/xyzct_8bit__mitosis.ome.zarr
napari --plugin napari-ome-zarr https://s3.embl.de/ome-zarr-course/data/ZARR/$USER/xyz_8bit_calibrated__fib_sem_crop.ome.zarr
Optional: visualise local OME-Zarr data in the same way:
napari --plugin napari-ome-zarr ~/data/ZARR/xyzct_8bit__mitosis.ome.zarr
Optional: visualise big remote OME-Zarr data:
napari --plugin napari-ome-zarr https://s3.embl.de/i2k-2020/platy-raw.ome.zarr
Note that compared to BigDataViewer, there are more delays with Napari.
Fiji/MoBIE
- Run Fiji with MoBIE
- Open OME-Zarr from S3 in BigDataViewer:
[ Plugins › BigDataViewer › OME ZARR › Open OME ZARR From S3... ]
S3 URL
: Choose one of the above example URLs- Log chunk loading
- Examine the IJ Log window to see:
- image scale levels
- transformations from data space to global space
- One OME-Zarr URL may contain multiple images, you can toggle them on and off:
- BDV: Press
P
and use theactive
checkbox- Examine multi-scale chunk loading:
[ Fiji > Window > Console ]
- Move around to load different chunks, e.g.:
- BDV:
Mouse scroll
to move along the current viewing axis- BDV:
Shift x
to view along the x-axis.- Zoom in to load higher resolution data:
- BDV:
Arrow up
Neuroglancer
- Open an image:
- Go to https://neuroglancer-demo.appspot.com/
- On to top right in
Source
, enter, e.g.,zarr://https://s3.embl.de/i2k-2020/platy-raw.ome.zarr
(replace the part behindzarr://
with your URL.- Press Enter (multiple times).
- Navigate around in the sample
- Zooming seems to require pinching on a trackpad
- Sharing views:
- The URL in your browser adapts to your current view
- Copy and paste the URL to share a view with a collaborator
- For example, try this one
vizarr
Open Google Chrome on BAND (for some reason vizarr does not work with Firefox on BAND). Google Chrome can be found under the Applications menu at the top left corner of the screen:
[Applications > internet > Google Chrome]
- To visualise a self-created OME-Zarr via vizarr, replace the $USER in the following link with your user name, copy-paste the link into the Google Chrome’s search bar and press enter:
https://hms-dbmi.github.io/vizarr/?source=https://s3.embl.de/ome-zarr-course/data/ZARR/$USER/xyzct_8bit__mitosis.ome.zarr
- Note: you can find your user name by entering
echo $USER
in the BAND terminal.- Optional: visualise the following in the same way:
- 3D EM data: https://hms-dbmi.github.io/vizarr/?source=https://s3.embl.de/ome-zarr-course/data/ZARR/$USER/xyz_8bit_calibrated__fib_sem_crop.ome.zarr
- A well from an HCS plate: https://hms-dbmi.github.io/vizarr/?source=https://s3.embl.de/eosc-future/EUOS/testdata.zarr/A/1
OME-Zarr segmentation
Segment remotely located OME-Zarr data without explicitly downloading it.
Show activity for:
ZarrSeg
Examine the dataset that is to be segmented:
mc tree -d 2 s3/ome-zarr-course/data/ZARR/$USER/23052022_D3_0002_positiveCTRL.ome.zarr
mc cat s3/ome-zarr-course/data/ZARR/$USER/23052022_D3_0002_positiveCTRL.ome.zarr/0/.zarray
Also view the data
napari --plugin napari-ome-zarr https://s3.embl.de/ome-zarr-course/data/ZARR/$USER/23052022_D3_0002_positiveCTRL.ome.zarr;
Perform threshold segmentation on each channel
zseg threshold -r -m otsu -c 1 -ch 0 -n otsu-c1-ch0 --colormap viridis ome-zarr-course/data/ZARR/$USER/23052022_D3_0002_positiveCTRL.ome.zarr;
In this command, the
-r
flag ensures that the input path is searched at the s3 bucket. The-m
option specifies the thresholding algorithm, which in this case is the Otsu algorithm. The-c
is a coefficient that is multiplied with the found threshold value to get the effective threshold. The-ch
species the channel 0 for segmentation. The-n
option specifies the name of the label path created.Now also segment the other channel:
zseg threshold -r -m otsu -c 1 -ch 1 -n otsu-c1-ch1 --colormap viridis ome-zarr-course/data/ZARR/$USER/23052022_D3_0002_positiveCTRL.ome.zarr;
Note that the
-ch
argument has been changed.Have a look at the segmented data
napari --plugin napari-ome-zarr https://s3.embl.de/ome-zarr-course/data/ZARR/$USER/23052022_D3_0002_positiveCTRL.ome.zarr;
Apply mathematical morphology to the label image
zseg postprocess -r -m binary_opening -f 1,1 -l otsu-c1-ch1 --colormap viridis ome-zarr-course/data/ZARR/$USER/23052022_D3_0002_positiveCTRL.ome.zarr;
Here the
-m
specifies the postprocessing method; the-f
determines the footprint shape. Depending on the shape of the input data, it can be 2 or 3-dimensional. The-l
can be used to decide on the name of the label image, that is subjected to the postprocessing.Now examine the OME-Zarr data:
mc tree -d 2 s3/ome-zarr-course/data/ZARR/$USER/23052022_D3_0002_positiveCTRL.ome.zarr
ome_zarr info https://s3.embl.de/ome-zarr-course/data/ZARR/$USER/23052022_D3_0002_positiveCTRL.ome.zarr
Also visualise the data:
napari --plugin napari-ome-zarr https://s3.embl.de/ome-zarr-course/data/ZARR/$USER/23052022_D3_0002_positiveCTRL.ome.zarr;
Assessment
Follow-up material
Recommended follow-up modules:
Learn more: